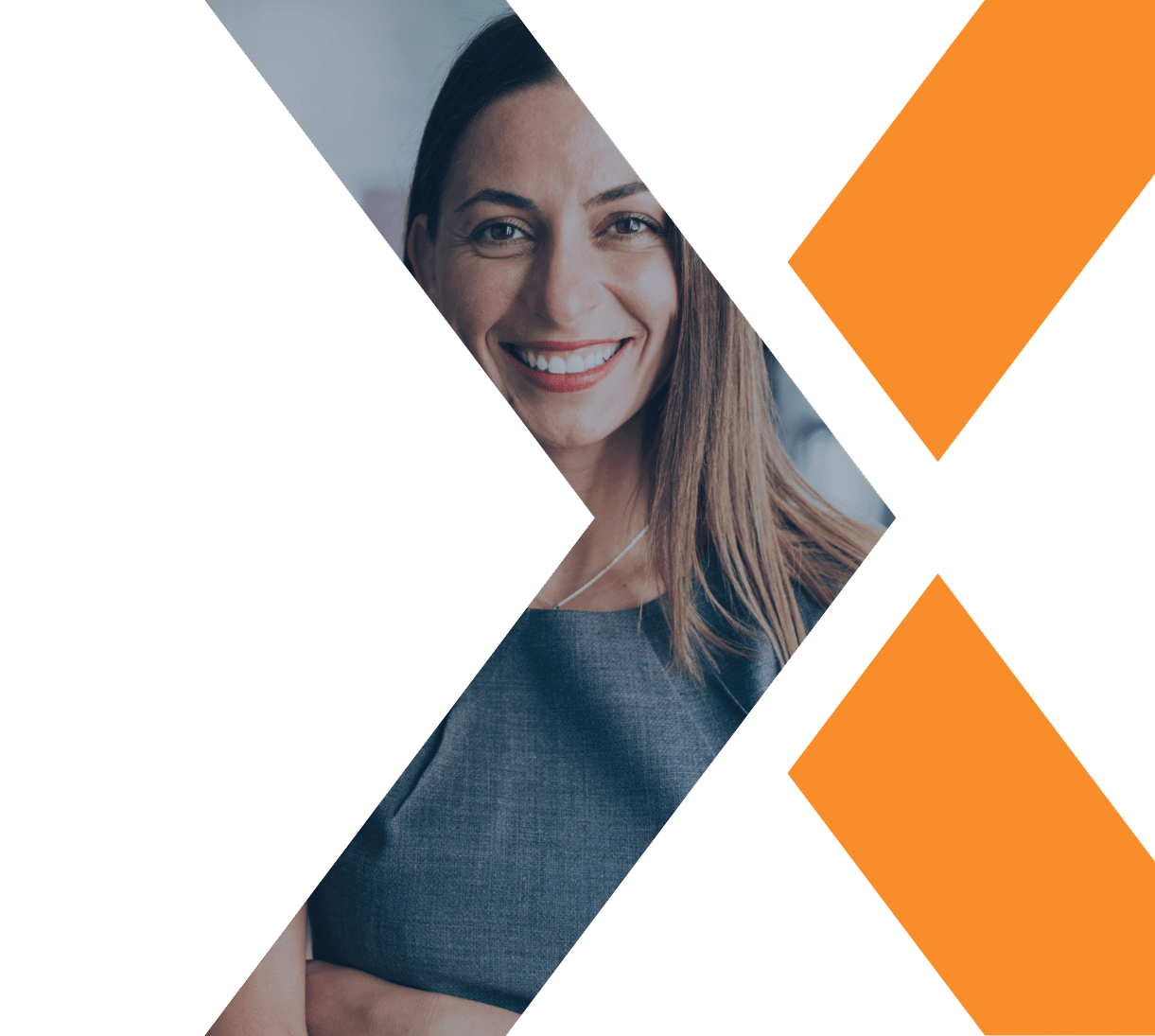
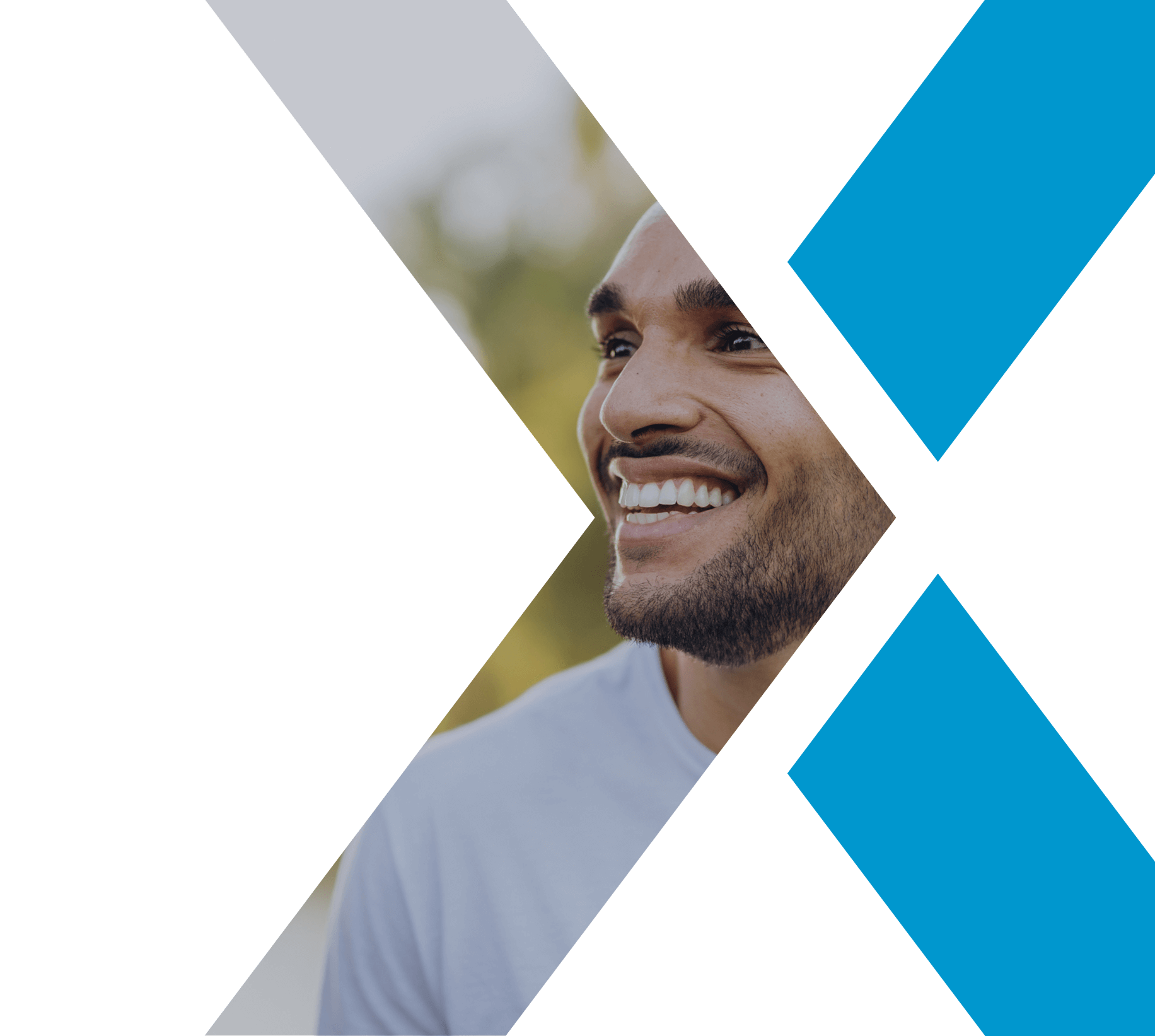
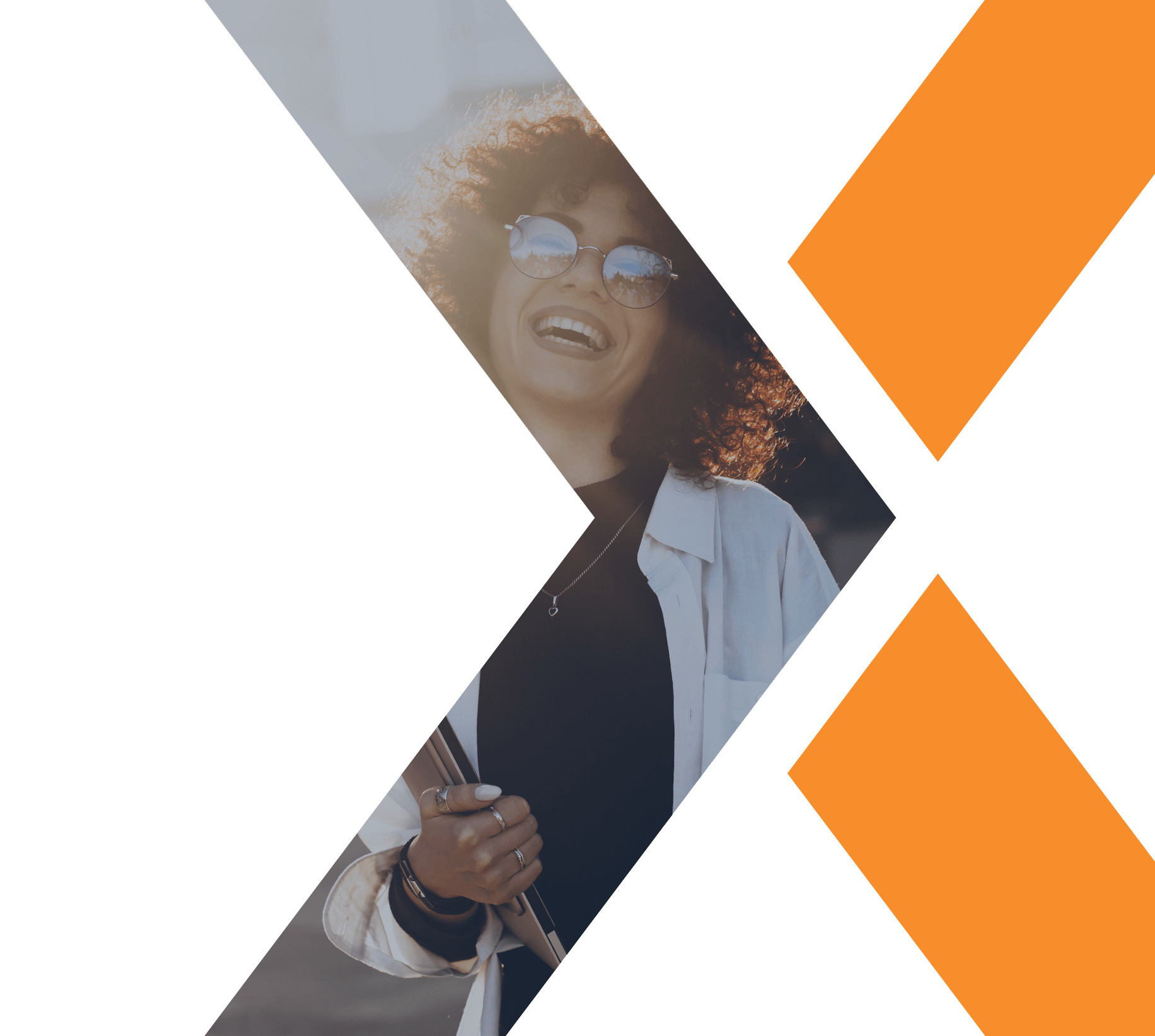
KI-basierte Recruiting-Technologie
Menschen und Jobs besser verbinden
Stärken Sie Ihre Personalbeschaffung, beschleunigen Sie Ihr Business Development und verbessern Sie das Talent Management mit unserer KI-basierten Recruiting-Technologie.
Mehr erfahrenJob Description API ist jetzt verfügbar!
Job Description API – Der KI-Schreibassistent der nächsten Generation
Erweitern Sie die Möglichkeiten Ihrer Plattform mit einem Schreibassistenten, der nicht nur detaillierte Stellenbeschreibungen erstellt, sondern den Nutzern auch Inspirationen zu relevanten Skills liefert. Unterstützt durch generative KI und Textkernels Skills Intelligence.
Mehr erfahrenDie Zukunft des Lebenslaufs parsing
DIE ZUKUNFT DES CV PARSINGS
Eine Parsing-Lösung, die den robusten, angereicherten proprietären Parser von Textkernel mit ChatGPT von Open AI kombiniert und so eine beispiellose Genauigkeit und grenzenlose Flexibilität bei der Datenextraktion bietet. Erschließen Sie neue Möglichkeiten in der Talentakquise.
Mehr erfahrenTalente und Arbeitsplätze besser verbinden
Unsere KI-basierten Lösungen optimieren das Recruiting und sorgen für eine effizientere Kandidatenauswahl. Erhalten Sie wertvolle Informationen und treffen Sie datengestützte Entscheidungen. Reduzieren Sie manuelle Aufgaben und bleiben Sie im Wettbewerb um Talente ganz vorne.
Parser
Die meistgenutzte Parsing-Engine der Branche für Genauigkeit und Geschwindigkeit.
Source & Match
Wir setzen den Standard für Matching- und Sourcing-Genauigkeit.
Skills Intelligence
Skill-basierte Ansätze durch unsere leistungsstarken Taxonomien.
Labor Market Insights
Strukturierte Echtzeit-Daten für den privaten und den Arbeitsmarkt für 15 Schlüsselmärkte in Nordamerika, Europa und APAC.
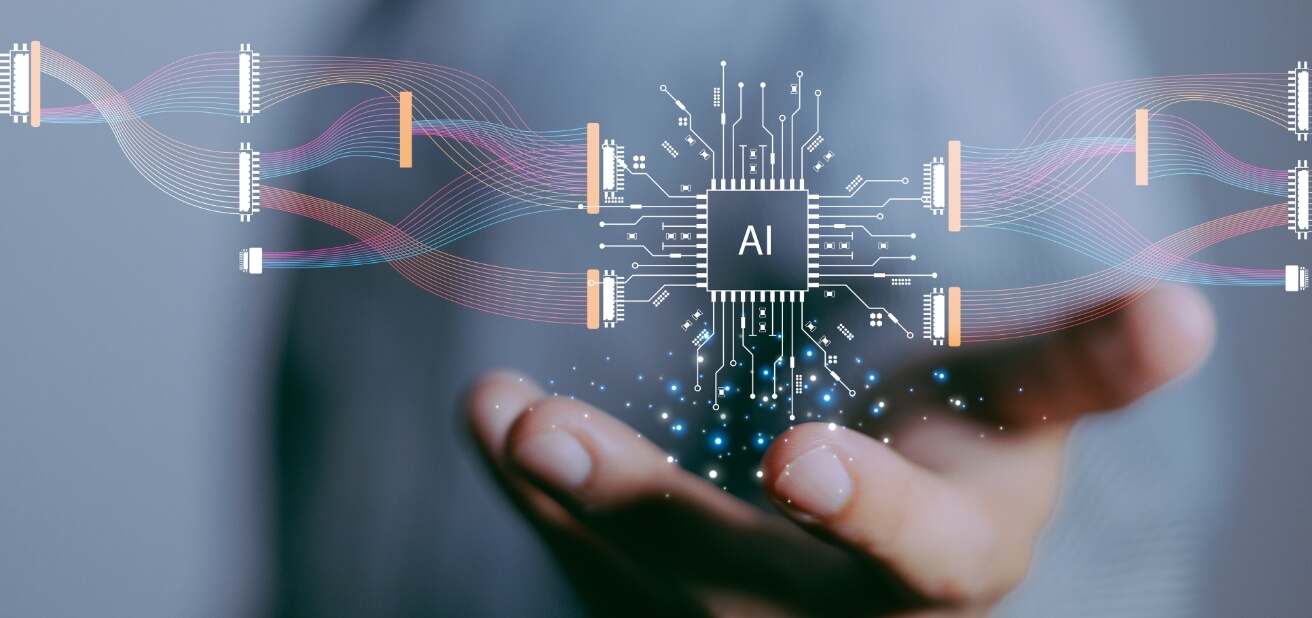
Verbessern Sie die Genauigkeit bei der recruitment und minimieren Sie Fehler bei der Dateneingabe durch automatische Datenextraktion.
Mehr erforschen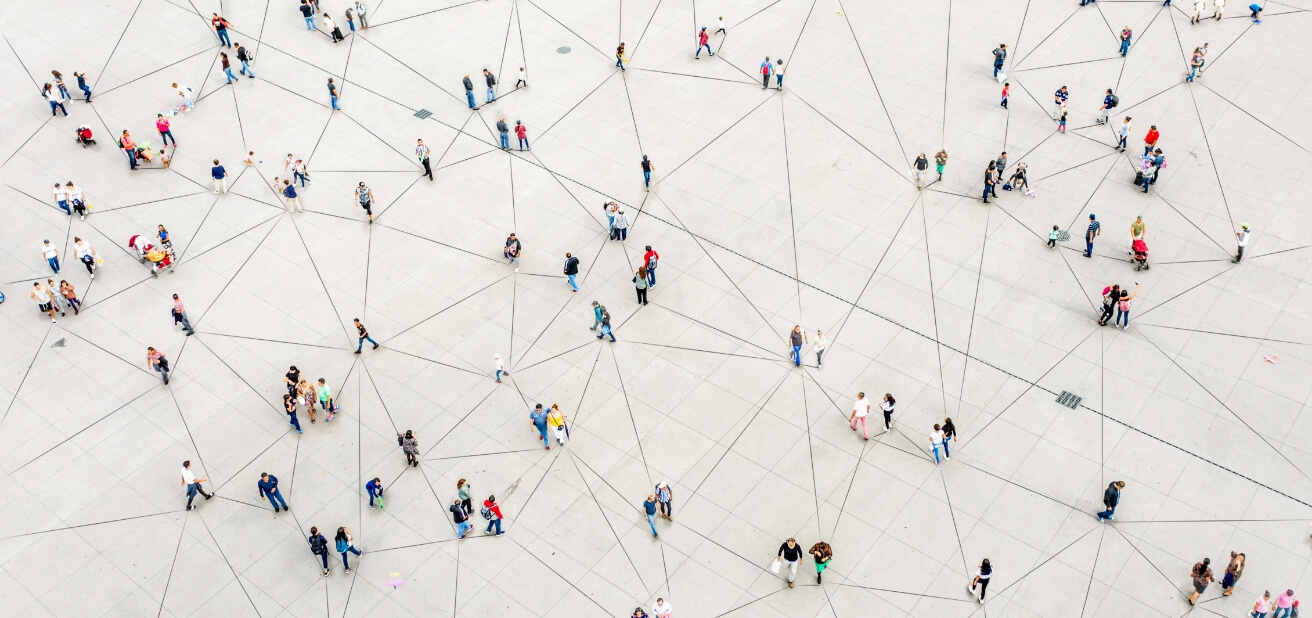
Verwandeln Sie Talentdaten in einen Wettbewerbsvorteil und ein wertvolles Kapital. Bringen Sie Menschen und Stellen mit der weltweit besten Matching Engine genauer zusammen.
Mehr erforschen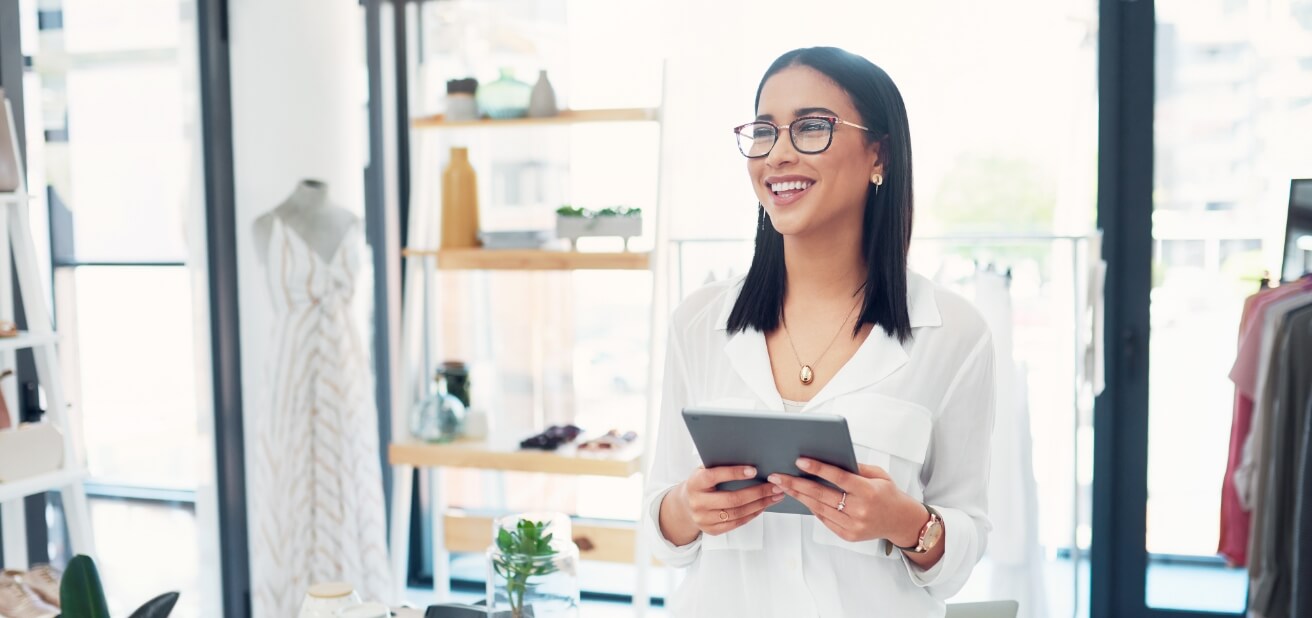
Unternehmen, Arbeitssuchende und Personalverantwortliche können künftige Qualifikationsanforderungen vorhersehen und effiziente Wege für die Weiterbildung, Umschulung, Einstellung und das Talentmanagement ermitteln.
Mehr erforschen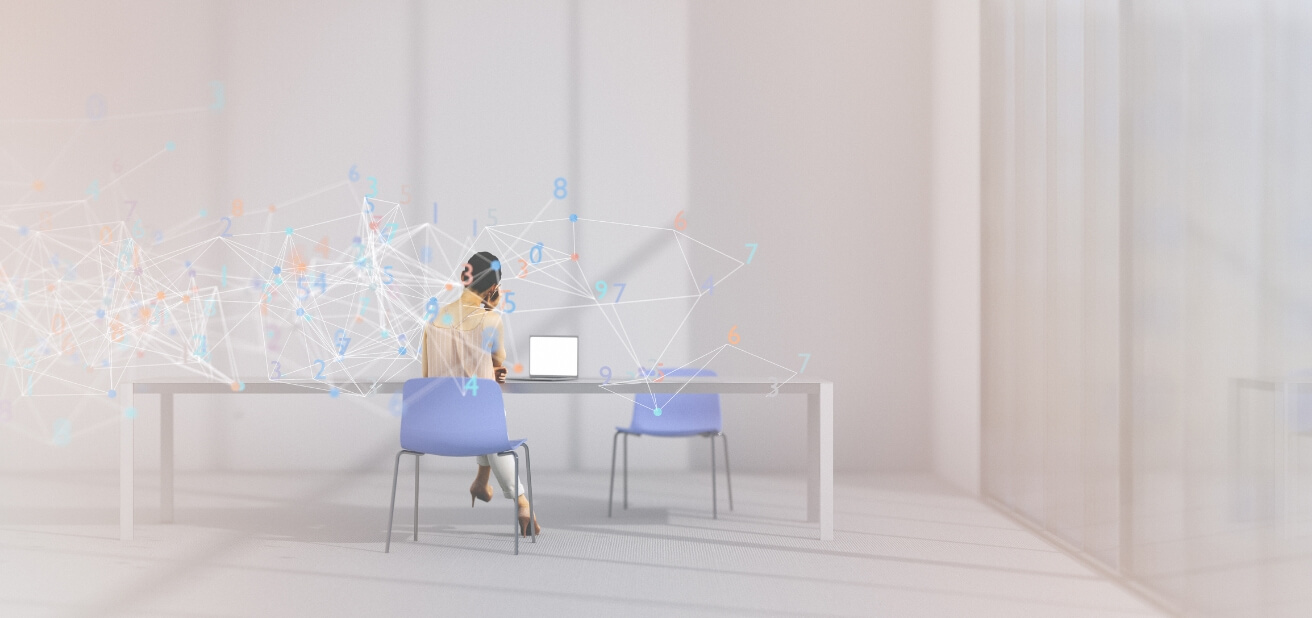
Arbeitsmarktinformationen für datengestützte Entscheidungsfindung. Führende Personaldienstleister, Fortune-500-Unternehmen und Regierungen weltweit vertrauen darauf.
Mehr erforschenErfolgsgeschichten von Kunden
Lesen Sie unsere neuesten Berichte über führende Unternehmen, die ihre recruitment- und -verwaltungsprozesse umgestalten.
Warum Textkernel?
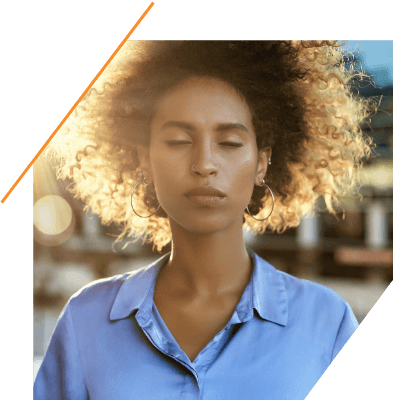
Wir sind Ihr Partner, wenn es darum geht, Menschen und Jobs miteinander zu verbinden
Als weltweit führender Anbieter von KI-gestützten Talentmanagement-Lösungen treibt Textkernel seit über 20 Jahren Effizienzsteigerungen in der HR-Tech-Branche voran. Unsere innovativen Technologien unterstützen mehr als 2.500 HR-Softwareanbieter, führende Unternehmen, Jobbörsen, Regierungsorganisationen und Analysten dabei, intelligenter zu arbeiten, schneller zu innovieren und sich in einem wettbewerbsintensiven Markt erfolgreich zu positionieren.
Wir sind führend in KI-Technologien für Recruiting und HR. Unsere leicht integrierbaren Lösungen verschieben die Grenzen dessen, was technologisch möglich ist.
Wir bieten maßgeschneiderte Lösungen weltweit an und bedienen 2.500 Kunden und Partner auf zahlreichen internationalen Märkten. Viele unserer Kunden sind selbst Marktführer und können die Effektivität unserer Lösungen bezeugen.
Für uns haben Datensicherheit und Datenschutz höchste Priorität. Daher wenden wir strenge Verfahren an im Umgang mit Kundendaten. Wir halten uns an die weltweite KI-Gesetzgebung, einschließlich ISO 27001, SOC II, GDPR-Zertifizierung und die europäische KI-ACT.
Unsere Technologie vereinfacht Prozesse und ermöglicht es Ihnen, sich auf das Wesentliche zu konzentrieren: die richtigen Menschen mit den richtigen Jobs zu verbinden. Wir bekämpfen Vorurteile bei Personalentscheidungen und fördern einen transparenteren Arbeitsmarkt, indem wir unsere Modelle nach strengen Grundsätzen für eine verantwortungsvolle KI entwickeln.
Wir sind einzigartig positioniert, um unsere Lösungen nahtlos in Ihr bestehendes HR-Technologie-Ökosystem zu integrieren. Unsere über 100 ATS- und HR-Software-Integrationen sprechen für sich.
FÜHRENDE UNTERNEHMEN VERTRAUEN AUF UNS
Abonnieren Sie unseren Newsletter und verpassen Sie nichts mehr!
Möchten Sie immer auf dem Laufenden bleiben zu den neuesten Entwicklungen in der Recruiting-Technologie? Klicken Sie auf den Button unten und verpassen Sie keine Updates.